SecAppDev 2023 lecture details
Security engineering for machine learning
How can the adoption of machine learning introduce systematic risk into our applications? This session discusses the results of applying architectural risk analysis to identify the top risks in engineering ML systems.
Monday June 12th, 09:15 - 10:30
Room Lemaire
Download handoutsAbstract
Machine Learning (ML) appears to have made impressive progress on tasks such as image classification, machine translation, and autonomous vehicle control. The surrounding hype and almost magical status of deep learing further drives the adoption of ML, often in a haphazard fashion. But can the adoption of ML introduce systematic risk into our applications?
Our research at the Berryville Institute of Machine Learning (BIIML) focuses on understanding and categorizing ML security engineering risks at the design level. In this session, we apply architectural risk analysis to ML systems and discuss the top risks that have been identified.
Key takeaway
The results of an architectural risk analysis (sometimes called a threat model) of ML systems, including the top five (of 78 known) ML security risks
Content level
Keynote
Target audience
All SecAppDev participants
Prerequisites
None
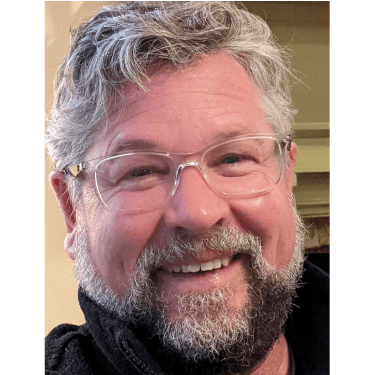
Gary McGraw
CEO, Berryville Institute of Machine Learning
Expertise: Software security, machine learning security, security engineering
Related lectures
Attacks against machine learning pipelines
Introductory lecture by Davy Preuveneers in room West Wing
Wednesday June 14th, 09:00 - 10:30
This session will explore various attacks against machine learning pipelines and their life cycle, present countermeasures and discuss best practices to make your ML models more robust in adversarial settings.
Key takeaway: ML adds value to applications but also increases the attack surface, imposing a holistic approach to secure the ML pipeline and lifecycle
The security model of the web
Introductory lecture by Philippe De Ryck in room Lemaire
Monday June 12th, 11:00 - 12:30
In this session, we explore how to leverage the fundamental security model of the web for security. We also explore complex attack patterns, such as CSRF, and how they impact even modern API-based applications.
Key takeaway: Understand how the browser reasons about web security, and how you can leverage this fundamental security model to secure your applications
Demystifying Zero Trust
Introductory lecture by Bart Preneel in room Lemaire
Wednesday June 14th, 09:00 - 10:30
We discuss the principles of zero trust and explain how it can be implemented. We also discuss how we can build up trust in devices, software and hardware components.
Key takeaway: Understand whether zero trust is useful for your organization or system. Reflect on which products and services you trust and why